Exploring the Impact of AI in R&D: Unveiling New Opportunities and Overcoming Challenges
Research and Development (R&D) is the backbone of innovation, prompting new breakthroughs across industries. In the past, the R&D process primarily relied on manual data collection and hypothesis testing, leaving room for both human and systematic error. However, with the rise of digital technology, the R&D landscape has rapidly transformed. Particularly, Artificial Intelligence (AI) has become a key player in R&D, providing new opportunities for innovation and paving the way for new discoveries.
AI has been at the forefront of this transformation. Since the late 20th century, AI has increasingly evolved to harness its potential in R&D. It can handle vast datasets, something that is prevalent in modern R&D processes. Traditional data processing methods are often inadequate in understanding such volumes of complex data. This is where AI, especially Machine Learning (ML) algorithms, can shine. By processing and learning from data, AI systems can analyse patterns, make predictions, and suggest innovative solutions.
One prime example of AI’s potential in R&D is its application in drug discovery in the pharmaceutical industry. AI’s ability to analyse molecular structures and predict biological activity of chemicals has significantly accelerated the discovery process, leading to faster and potentially more effective drug solutions.
However, integrating AI into R&D comes with its own set of challenges. Data privacy concerns have arisen as AI requires massive amounts of data to function optimally. Ensuring data is not misused or mishandled is of paramount importance. There is also the question of trust in these AI systems. While they can process vast amounts of data at unparalleled speeds, they are also susceptible to errors when fed inaccurate or biased data.
Despite these challenges, there is no denying that AI will continue to exert a growing influence on R&D in many industries. As with all powerful tools, it comes with its own set of challenges that need to be addressed to truly harness its potential and unlock the many benefits for more accelerated innovation.
Historical Context
The early applications of AI in research can be traced back to the 1960s when the first AI programs were developed to assist in performing complex reasoning and problem-solving tasks. However, it wasn't until the 1990s that AI started to gain traction in R&D. During this period, AI was primarily used in data mining and analysis to make sense of large datasets. But with the development of more sophisticated machine learning algorithms, AI's involvement in R&D has evolved. In recent years, AI has found myriad applications in R&D, including drug discovery, genetic research, material science, and nanotechnology. Its ability to analyse vast data sets and identify patterns has significantly accelerated the research process, helping researchers make discoveries that would otherwise have been impossible.
One recent example of AI's potential in R&D is the development of a deep learning algorithm that can predict the conformation of small molecules with high accuracy. This algorithm, developed by researchers at the University of Cambridge, could revolutionise drug discovery by helping researchers predict the efficacy of potential drug compounds before they go through the costly and time-consuming process of synthesis and testing.
Overall, AI's involvement in R&D has come a long way since its early days. As the technology continues to evolve, we can expect to see even more transformative applications in the years to come. However, as with any transformative technology, there will be challenges to overcome. Researchers, policymakers, and industry leaders must work together to ensure that AI is used responsibly and ethically, and that its benefits are enjoyed by all.
Opportunities Presented by AI in R&D
The opportunities presented by AI in R&D are vast. From enhanced data analysis to speeding up prototyping and predictive analysis, AI has the potential to revolutionise the way researchers work.
Enhanced Data Analysis:
One of the most significant benefits of AI in R&D is its capability to handle vast data sets. Traditional data processing methods fall short when it comes to analysing such data meaningfully. However, AI, especially machine learning algorithms, can learn from data, identify patterns, and even suggest innovative solutions that might not be immediately apparent to human researchers. A prime example of this is drug discovery in the pharmaceutical industry, where AI's capability to analyse molecular structures and predict their biological activity has significantly sped up the discovery process, leading to faster and potentially more effective drug solutions.
Speeding Up Prototyping:
AI-driven simulations have significantly reduced the time and resources spent on prototyping. In industries like aerospace or automotive design, virtual AI simulations can predict how a particular model will perform under certain conditions, long before a physical prototype is created. In a real-world example, a major car manufacturer saved millions by using virtual simulations during the initial design phase to identify flaws and optimise performance before production.
Predictive Analysis:
Another significant advantage of AI in R&D is its ability to forecast trends and potential outcomes in research. By analysing data and identifying patterns, AI systems can predict the likelihood of a particular event or outcome. For example, in the healthcare industry, AI algorithms can analyse patient data to predict which individuals are at risk of developing certain diseases. This predictive analysis can lead to earlier interventions and more effective treatment options.
Collaborative Robots and AI Tools:
The next frontier in AI and R&D is the role of AI in enhancing human-machine collaboration. Collaborative robots, or cobots, are designed to work alongside human researchers to complete tasks collaboratively. A renowned lab in Switzerland is already using cobots to test the strength of new materials. The lab's cobots are programmed to apply specific forces to samples, while the researchers observe and record data, enabling them to focus on data analysis and interpretation.
Customisation and Personalisation in R&D:
Finally, AI has the potential to improve customisation and personalisation in R&D. By tailoring research based on AI-driven insights, researchers can develop products that better meet users' needs and preferences. For example, a tech company has used AI personalisation techniques to develop earbuds that adapt to the wearer's unique hearing profile, resulting in a more personalised listening experience.
AI's potential in R&D is vast, and the benefits of enhanced data analysis, faster prototyping, predictive analysis, collaborative robots and AI tools, and customisation and personalisation cannot be overstated. However, challenges such as data privacy, trust, ethics, skill gaps, and cost cannot be ignored. Addressing these challenges is critical for researchers to truly harness AI's potential and drive innovation.
Challenges Facing AI Integration in R&D
Artificial Intelligence (AI) is quickly becoming an indispensable tool in research and development (R&D). From accelerating the drug discovery process to simulating product prototypes, AI has proven to be a game changer. However, AI integration is not without its challenges. In this blog, we will explore the top challenges facing AI integration in R&D and how they are being addressed.
Data Privacy and Security
AI systems require vast amounts of data to function optimally. This poses serious risks for data privacy and security. In an R&D firm, a data breach could lead to the loss of valuable research findings, compromising the firm's competitive edge. Therefore, ensuring data privacy and security is of paramount importance. R&D firms should invest in robust data management systems and ensure all employees are trained in data privacy and security best practices.
Reliability and Trust
Reliability and trust are major concerns when it comes to AI in R&D. There is always a risk that an AI system will make a mistake, leading to inaccurate research findings. In an R&D project, this could be disastrous, potentially leading to significant setbacks and wasted resources. To address this risk, researchers must validate AI outcomes with traditional methods to ensure accuracy and consistency.
The Ethical Implications
The ethical implications of AI-driven research decisions cannot be overlooked. AI is solely designed to optimise outcomes based on previously collected data, whereas human researchers can take into account additional factors such as ethics, morality, and social impact. The controversial case of AI-driven medical research highlights this debate. The AI system, trained on a vast patient database, recommended more biopsies than human doctors. While the recommendations were optimised for higher cancer detection rates, there were concerns about the number of unnecessary biopsies and the associated risks. It is essential that AI systems are guided by human values and ethical principles to ensure their decisions are aligned with human values.
Skill Gaps and Training
As AI tools become increasingly sophisticated, the need for specialised skills in R&D is growing. There is a pressing need for researchers and scientists to be equipped with the knowledge to use these tools effectively. While some universities are offering AI courses, there is still a significant gap between skills demand and supply. Dedicated training programs can play a crucial role in addressing this gap. Companies can invest in training programs that bridge the AI skills gap and empower their employees with the necessary skills to work with AI successfully.
Cost Implications
The initial investment required for AI integration in R&D can be substantial. While AI can potentially save companies millions in the long run, the cost of technology and training can be a barrier to entry. Companies must evaluate the return on investment (ROI) of AI implementation before investing. According to a leading research institution, 60% of the initial cost of AI implementation is recouped within three years, and an AI-powered R&D department can yield a 40% increase in productivity. Therefore, companies must take a long-term view when weighing the cost implications of AI implementation.
AI integration in R&D presents significant opportunities but can also pose some challenges. Addressing these challenges requires close attention to data privacy and security, validation of AI outcomes, ethical considerations, skill development, and cost implications. However, the benefits of AI far outweigh these challenges. Companies that successfully integrate AI into their R&D processes stand to gain a significant competitive advantage. By investing in AI, companies can speed up research cycles, reduce costs, improve accuracy and productivity, and generate more innovative solutions. As AI continues to evolve, it is essential that we continue to address these challenges to realise its full potential.
The Future of AI in R&D: Expert Predictions
The future of AI in R&D is undeniably bright, with leading experts in the field predicting significant advancements in the coming years. Dr. Andrew Ng, a renowned expert in AI, predicts that within the next decade, 50% of all R&D tasks will be automated using AI. This is due to the increasing adoption of AI by industries such as healthcare, where it has already been used to create solutions that improve patient diagnoses, monitoring, and care. Additionally, AI is expected to revolutionise other industries such as agriculture, energy, and finance.
One potential innovation for AI in R&D is the development of "explainable AI" systems. These systems not only produce accurate results but also provide explanations as to how they arrived at those outcomes. This feature will be crucial, especially in highly regulated fields such as healthcare and finance, where transparency and accountability are critical.
However, challenges such as data privacy and trust in AI systems will need to be addressed, especially in highly sensitive research areas such as drug discovery and clinical trials. Experts such as Dr. Andre Esteva emphasise the importance of involving researchers and clinicians in the design, development, and validation of AI systems to ensure their trustworthiness.
Another challenge is the lack of experienced professionals in using AI tools effectively. Upskilling of researchers and scientists in advanced AI technologies is essential to maximise their potentials. Additionally, research institutions and organisations need to invest more resources in research and development to overcome hurdles posed by regulatory compliance and the initial cost of investment.
In conclusion, while AI in R&D presents several challenges, the potential benefits are enormous. Experts predict that, by 2030, industries that adopt AI in their R&D processes will leverage unprecedented speed, accuracy, and cost efficiency. It is, therefore, imperative that research institutions and organisations work to address the challenges and take advantage of the potential that AI presents in R&D to stay ahead of the competition.
Conclusion:
In conclusion, the impact of AI in R&D has been transformative in many ways. From drug discovery to virtual simulations, AI has enabled researchers to achieve outcomes that were previously unattainable. However, we must carefully balance the benefits of leveraging AI's potential with addressing the challenges it presents. The issue of data privacy and trust in AI systems are factors that must be carefully considered and addressed going forward. We must also ensure that the ethical implications of relying on machines for research decisions are thoroughly examined.
Moreover, there is a need for researchers and scientists to be equipped with the necessary knowledge to use these advanced AI tools effectively. Despite the challenges surrounding AI’s integration into R&D, the potential benefits in terms of cost savings, increased efficiency, and faster discoveries make the investment worthwhile.
As we move towards a world that places greater emphasis on digital technologies, there is no doubt that AI will continue to play an increasingly central role in R&D. However, it is essential that we as a society remain vigilant in addressing any potential issues surrounding AI, while taking full advantage of its tremendous potential. By doing so, we can continue to advance innovation across industries and achieve remarkable results for society as a whole.
Get in touch
If you would like to discuss any aspect of R&D Tax Credits or the Tax Cloud portal, feel free to contact our friendly expert team on 020 7360 4437 or send us a message.
- Submitting R&D tax claims since 2001
- Strong track record spanning 20+ years delivering R&D tax credit claims
- Over £70m claimed and counting
- Industry leading specialists
- We employ technical, costing and tax experts and tax experts
- Confident of delivering value to our clients, we offer our R&D tax services on a success fee-only basis.
Meet some of the team behind Tax Cloud
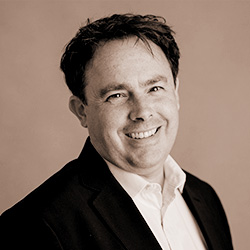
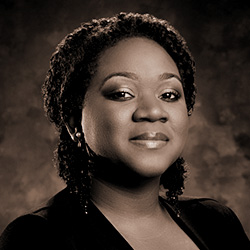
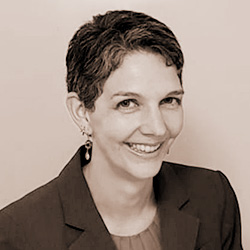
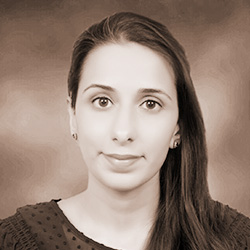